Eyes, JAPAN
Generative Streaming : Will the way we send things over the internet completely change?
zeke
Year after year, global internet traffic continues to grow with no signs of stopping, as more and more aspects of our daily lives become interconnected with the internet. From streaming our favorite shows to managing smart home devices, we are constantly online. In response to this increasing demand, significant improvements in network capabilities, data compression, and topologies like content delivery networks (CDNs) have been essential tools for making streaming and data transmission over the internet more efficient. However, we are approaching the limits of data compression, as there is only so much redundancy that can be removed using traditional compression techniques without compromising the quality. Like many of our other problems, engineers have chosen the very reliable method of “just use AI” once again as the solution, giving birth to Generative Streaming. This blog will explain how Generative streaming may change the entire internet architecture and hopefully we will never have to wait for a video to buffer again!
What is Generative Streaming?
We have seen the terrifying power of recent generative models that have the ability to generate very realistic content of anything we want, convincing enough to trick our grandparents into believing anything. Generative streaming uses this great power, responsibly of course, and is actually quite self-explanatory. As the name suggests, it fundamentally works by generating the content ourselves! So in the distant future we may be generating the content on our own devices in real time! Imagine generating the ‘Avengers Endgame’ movie on your tiny phone, it will be as easy as snapping your fingers; no pun intended.
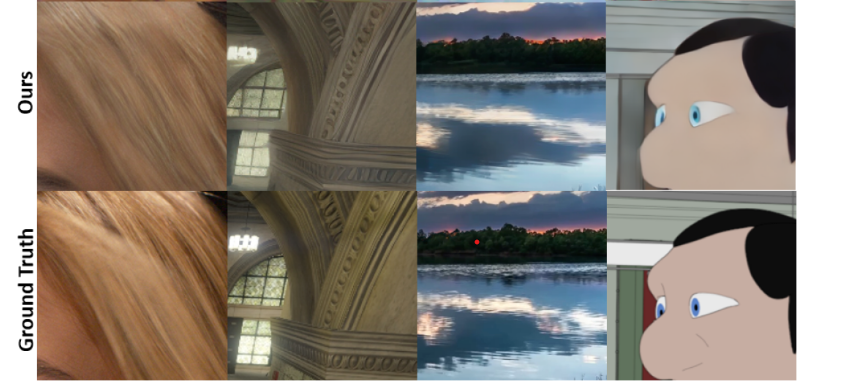
The ‘Ours’ images shows the Promptus generated content in relation to the Ground Truth. The generated content still retains a lot of the high level features and looks very similar at first glance.
Generative streaming pipeline
Without going into too much technical detail, a generative streaming pipeline still follows a standard encoder decoder flow used in communication networks today. Instead of encoding the data with normal compression techniques, for generative streaming we encode the data into compact semantic representations. These representations are smaller in size and hold the essential information of the content. Some researchers have even compressed these representations even more by utilizing standard compression techniques like quantization. They can come in many forms depending on the modal being sent, for example, text may be represented as embeddings, images might use features extracted through convolutional layers, and videos could focus on transmitting only residuals (the changes between frames). These representations are then sent to the receiver through standard networking protocols, where it will be decoded which in this case, means it will be passed to a generative model to generate the content.
Current shortcomings
It sounds simple enough right? Although in theory, the pipeline is not complicated, as of now it is quite an unexplored topic and there are significant hurdles to overcome.
Firstly, the limitations of current generative models right now, as expected, will still be a problem in generative streaming. Generative models still suffer from high computational cost and of course artifacts, which are visual errors in the output. Generating high-quality content in real time requires substantial processing power on the receiving device, far beyond what most consumer-grade devices are capable of today.
Secondly, the ability to retain the important information in the semantic representation. As of now there is no real metric or way to tell if a representation is capturing essential information of the content. This can call into question many control issues, especially in multi-user communication situations. If I send the same latent representation to 100 different people, will their model generate exactly the same content? If I send a video to someone, and they encode their generated version to send to someone else, after a few repetitions will the generated content still match the original?
Lastly is keeping the knowledge base of the generative model the same. If the models on the sender and receiver end have been trained on different data and thus have different knowledge bases, will it be able to generate the correct content? Of course we can train them together, but then how will we ensure that they stay updated?
Conclusion
There are still a lot of unanswered questions that have to be addressed in the domain before it becomes practically feasible. Although, there are already some researchers that have successfully employed the generative streaming idea for more specific tasks like video conferencing, showing the potential it has but achieving generality where any type of content can be sent is still quite far into the future. While of course all of these things will continue to improve, for now it makes generative streaming practically not feasible. Nevertheless, the vision of transmitting only semantic representations of data is promising and likely achievable within our lifetime
References :
Wu, Jiangkai & Liu, Liming & Tan, Yunpeng & Hao, Junlin & Zhang, Xinggong. (2024). Promptus: Can Prompts Streaming Replace Video Streaming with Stable Diffusion. 10.48550/arXiv.2405.20032.